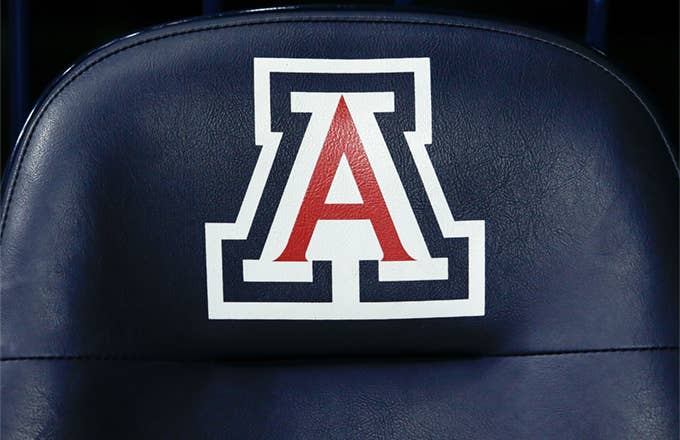
Researchers at the University of Arizona have been tracking the swipes of freshmen students’ ID cards to predict which of them are most likely to drop out.
Over the last three years, a professor of management information systems named Sudha Ram has been analyzing data gathered from the cards about how often students enter residence halls, libraries, and student recreation centers—as well as how frequently they make purchases.
"It’s kind of like a sensor that’s embedded in them, which can be used for tracking them," Ram explains in a University of Arizona press release. "It’s really not designed to track their social interactions, but you can, because you have a timestamp and location information."
The idea is that info about student habits on campus can tell researchers a lot about social behavior. Ram uses the data to create networks that map out which students interacted with each other, and how often those interactions took place. She says, "There are several quantitative measures you can extract from these networks, like the size of their social circle, and we can analyze changes in these networks to see if their social circle is shrinking or growing, and if the strength of their connections is increasing or decreasing over time."
By analyzing behavioral patterns, Ram is able identify trends like shrinking social circles to identify which students are at high risk of dropping out.
"Of all the students who drop out at the end of the first year, with our social integration measures, we're able to do a prediction at the end of the first 12 weeks of the semester with 85 to 90 percent recall," Ram says. "That means out of the 2,000 students who drop out, we're able to identify 1,800 of them."
The hope is that these students could then be paired with school advisers and receive assistance to get on track and avoid dropping out. “As early as the first day of classes, even for freshmen, these predictive analytics are creating highly accurate indicators that inform what we do to support students in our programs and practice," says Angela Baldasare, assistant provost for institutional research.
Of course, the method of tracking student information has raised concerns about privacy. To help alleviate these concerns, the data has been anonymized, so Ram can't personally identify individual students by name. She explains, "Almost every prediction we make is personalized, without knowing who the individual is."
So far, the information has been used for research purposes, but Ram says she hopes her techniques will one day be used by universities to supplement the predictions that they're already making based on demographics and other data.
"Student retention is something that's been studied for the last 30 or 40 years, but we never had the ability to track people's behavior and movement and extract their social integration patterns," she says. "We have also made great strides in developing machine learning and large-scale network analysis methods that help in analyzing such spatio-temporal data."